Geographically Varying Relationship of Dengue Incidence with Climate Factors in Malaysia
DOI:
https://doi.org/10.37934/scsl.1.1.3851bKeywords:
Geographically Weighted Regression, Generalized Linear Model, dengue, stationarity, spatial autocorrelationAbstract
Dengue, a mosquito-borne arboviral disease transmitted by Aedes mosquitoes, has been a growing public health burden. Since dengue data is collected spatially, non-stationarity in the data series is possible. Therefore, we employ a Geographically Weighted Regression (GWR) model to ascertain the correlation between dengue incidence and location-specific predictor factors. Nevertheless, because GWR is based on conventional least squares, it has several drawbacks, including the inability to consider non-continuous variables, non-linear relationships, or non-Gaussian error distributions. Therefore, this study suggests a hybrid model called a Generalised Geographically Weighted Regression (GGWR), which combines Geographically Weighted Regression and a Generalised Linear Model (GLM) to address these flaws. This study aims to examine the influence of climate factors on the increase of dengue incidence in Malaysia and to establish their relationship using the proposed GGWR model. Stationarity and spatial autocorrelation assumptions will be evaluated using the Breusch-Pagan and Moran's tests, respectively, while normality will be assessed through the Jarque-Bera test. In addition, since the data series shows a problem of overdispersion, a negative binomial is proposed. Compared to GLM, the GGWR model offers a superior fit based on the minimum Akaike information criterion. This study also found that the GGWR negative binomial performed better than the GGWR Poisson regression based on the mean square errors in dealing with the overdispersion problem. The GGWR and GLM models demonstrated that climate factors substantially influence dengue incidence. The proposed GGWR model provides a more robust framework for understanding the spatial variability in dengue outbreaks, offering valuable insights for policymakers to devise more effective preventive strategies to mitigate the spread of the disease. The contributions of the study lie in developing and applying the GGWR model, which addresses key limitations of traditional GWR and GLM approaches, providing a more refined tool for analysing spatially varying relationships in public health data.
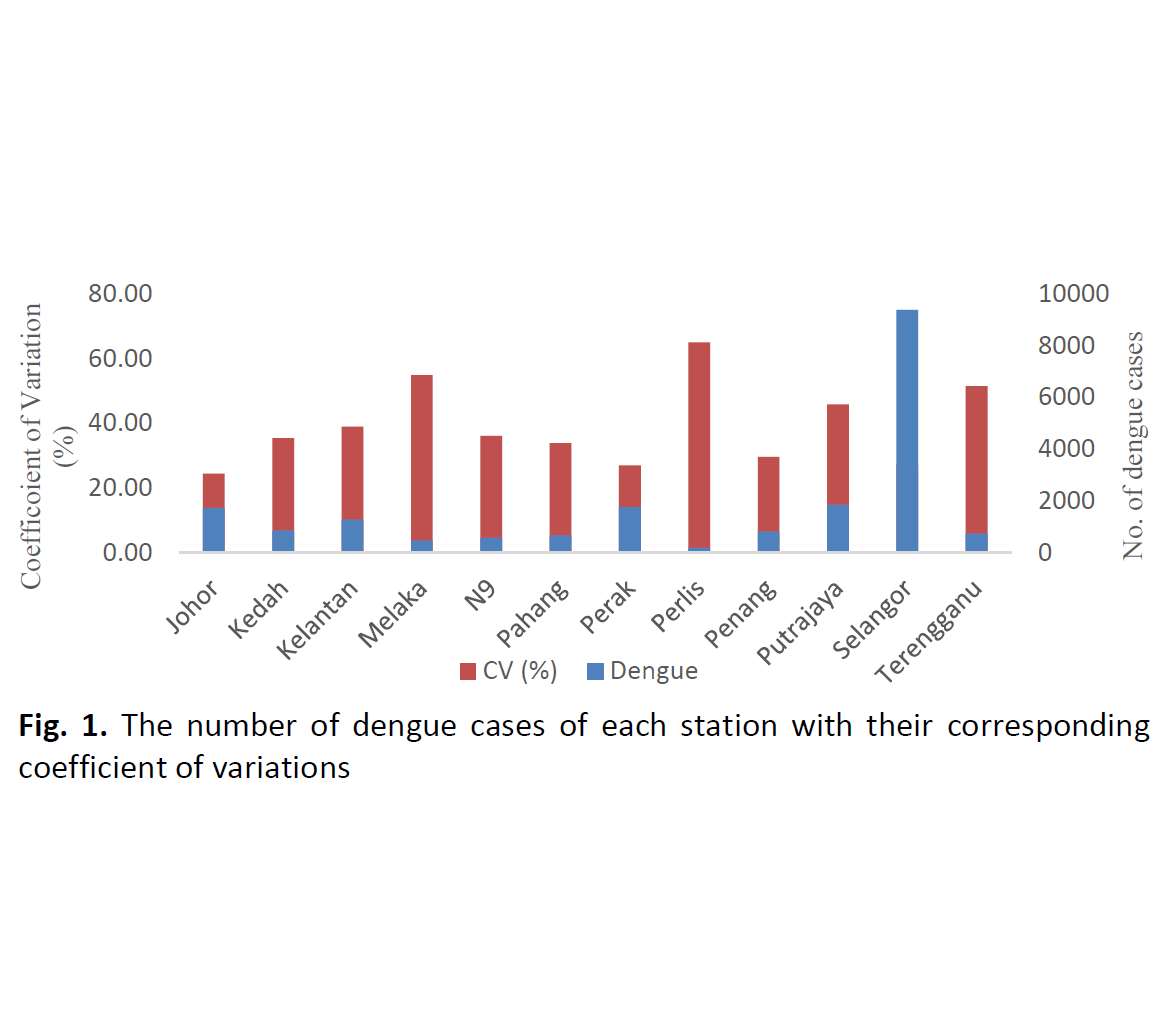